#118 – Effective Adoption of AI in the Enterprise – with Anindya Ghose
BOUNDARYLESS CONVERSATIONS PODCAST - EPISODE 118
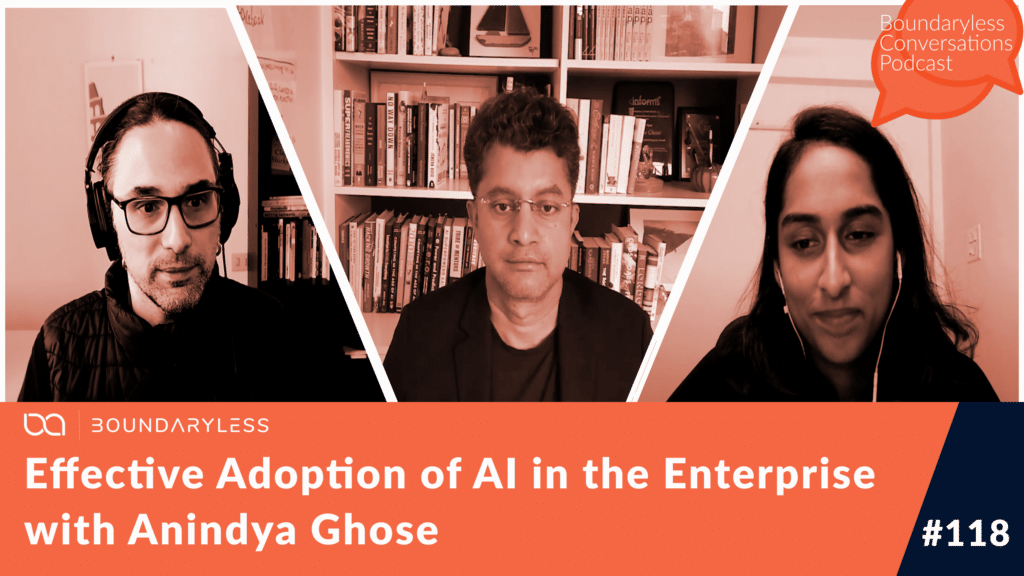
#118 – Effective Adoption of AI in the Enterprise – with Anindya Ghose
What does it take for organizations to truly harness the power of AI – not just as a buzzword, but as a transformative force for strategy, culture, and execution? In this episode, Anindya Ghose – Professor at NYU Stern, and one of the world’s leading voices at the intersection of AI, economics, and platforms – joins us to explore what it means to adopt AI intentionally and ethically.
Anindya presents his “House of AI” framework to cut through the AI hype. This grounded, end-to-end framework helps organizations align their data, models, and ethics for meaningful impact.
He also shares his insights on how AI is shifting the nature of management itself – and why the true differentiator in an AI-enabled world will be people, not technology.
Youtube video for this podcast is linked here.
Podcast Notes
In this episode, we go beyond surface-level AI conversations to explore what it really means to embed it within an organization – not just as a tool, but as a force that reshapes how decisions are made, work gets done, and leadership is defined.
Anindya is a globally recognized AI advisor who has transformed the journey of over 60 companies worldwide. As the author of Tap and Thrive, he also brings a rare blend of corporate insight and academic depth, helping us break down how AI can elevate both business and human potential.
In our conversation, we explore how AI is pushing organizations to rethink core assumptions, what are the cultural and structural implications of AI, how does AI take shape in organizational structure, and why it’s important to also see AI as a powerful positive force.
This is not an episode to miss, as we explore the tensions around AI and how they can be turned into meaningful opportunities.
Key highlights
👉 AI transformation starts with intentionality, requiring organizations to align their data, business context, and strategic goals before deploying any tools or models.
👉 Data engineering is 70% of the work, and without clean, structured data, even the most advanced AI will fail to deliver meaningful results.
👉 The House of AI framework provides a step-by-step path that moves from data readiness to modelling and ends with ethical, transparent storytelling.
👉 AI adoption requires organizations to answer four core questions – descriptive (what happened), predictive (what will happen next), causal (why), and prescriptive (what should we do).
👉 AI is evolving from a decision-support tool into a force of production, fundamentally altering how workflows operate and how organizations create value.
👉 Fairness can be built into AI through data debiasing, but ethics largely remains a human responsibility shaped by incentives, governance, and cultural values.
👉 Competitive advantage will depend less on technology access and more on the people, mindsets, and ability to act on insights quickly and responsibly.
This podcast is also available on Apple Podcasts, Spotify, Google Podcasts, Soundcloud and other podcast streaming platforms.
Topics (chapters):
00:00 Effective Adoption of AI in the Enterprise – Intro
00:58 Introducing Anindya Ghose
02:44 House of AI Framework – Getting Real with AI
10:47 Intentionality of Adopting AI
13:38 Changing the conversation around AI’s Positivity
18:22 AI’s new regime in radical disruption
23:05 How is AI altering the culture of Management in Organizations?
29:25 Preparing for AI Dominated Organizations
32:47 The Future of Competition in an AI-Driven Market: Openness vs. Differentiation
38:47 How will Organizations Differentiate?
41:46 Is it a Winner Takes All Market
43:39 Breadcrumbs and Suggestions
To find out more about his work:
- Anindya Ghose -Wikipedia
- Anindya Ghose – Website
- Anindya Ghose – Linkedin
- Anindya Ghose – Twitter (X)
Other references and mentions:
The podcast is recorded by 5 March 2025.
Get in touch with Boundaryless:
Find out more about the show and the research at Boundaryless at https://boundaryless.io/resources/podcast
Twitter: https://twitter.com/boundaryless_
Website: https://boundaryless.io/contacts
LinkedIn: https://www.linkedin.com/company/boundaryless-pdt-3eo
In this episode, we go beyond surface-level AI conversations to explore what it really means to embed it within an organization – not just as a tool, but as a force that reshapes how decisions are made, work gets done, and leadership is defined.
Anindya is a globally recognized AI advisor who has transformed the journey of over 60 companies worldwide. As the author of Tap and Thrive, he also brings a rare blend of corporate insight and academic depth, helping us break down how AI can elevate both business and human potential.
In our conversation, we explore how AI is pushing organizations to rethink core assumptions, what are the cultural and structural implications of AI, how does AI take shape in organizational structure, and why it’s important to also see AI as a powerful positive force.
This is not an episode to miss, as we explore the tensions around AI and how they can be turned into meaningful opportunities.
Key highlights
👉 AI transformation starts with intentionality, requiring organizations to align their data, business context, and strategic goals before deploying any tools or models.
👉 Data engineering is 70% of the work, and without clean, structured data, even the most advanced AI will fail to deliver meaningful results.
👉 The House of AI framework provides a step-by-step path that moves from data readiness to modelling and ends with ethical, transparent storytelling.
👉 AI adoption requires organizations to answer four core questions – descriptive (what happened), predictive (what will happen next), causal (why), and prescriptive (what should we do).
👉 AI is evolving from a decision-support tool into a force of production, fundamentally altering how workflows operate and how organizations create value.
👉 Fairness can be built into AI through data debiasing, but ethics largely remains a human responsibility shaped by incentives, governance, and cultural values.
👉 Competitive advantage will depend less on technology access and more on the people, mindsets, and ability to act on insights quickly and responsibly.
This podcast is also available on Apple Podcasts, Spotify, Google Podcasts, Soundcloud and other podcast streaming platforms.
Topics (chapters):
00:00 Effective Adoption of AI in the Enterprise – Intro
00:58 Introducing Anindya Ghose
02:44 House of AI Framework – Getting Real with AI
10:47 Intentionality of Adopting AI
13:38 Changing the conversation around AI’s Positivity
18:22 AI’s new regime in radical disruption
23:05 How is AI altering the culture of Management in Organizations?
29:25 Preparing for AI Dominated Organizations
32:47 The Future of Competition in an AI-Driven Market: Openness vs. Differentiation
38:47 How will Organizations Differentiate?
41:46 Is it a Winner Takes All Market
43:39 Breadcrumbs and Suggestions
To find out more about his work:
- Anindya Ghose -Wikipedia
- Anindya Ghose – Website
- Anindya Ghose – Linkedin
- Anindya Ghose – Twitter (X)
Other references and mentions:
The podcast is recorded by 5 March 2025.
Get in touch with Boundaryless:
Find out more about the show and the research at Boundaryless at https://boundaryless.io/resources/podcast
Twitter: https://twitter.com/boundaryless_
Website: https://boundaryless.io/contacts
LinkedIn: https://www.linkedin.com/company/boundaryless-pdt-3eo
Transcript
Simone Cicero
Hello everybody and welcome back to the Boundaryless Conversations Podcast. On this podcast, we explore the future of business models, organizations, markets and society in our rapidly changing world. I am joined today by my usual co-host, Shruthi Prakash. And hello Shruthi.
Shruthi Prakash
Hello everybody.
Simone Cicero
And we are honored to welcome our guest for today, one of the leading world experts on digital platforms, data, business models, the intersection of AI and economics and consumer behaviours, Anindya Ghose. Hello, Anindya, it’s great to have you here.
Anindya Ghose
Thank you.
Thank you, Simone and Shruthi. Thanks for having me. Look forward to the conversation.
Simone Cicero
Thank you. Anindya is the Heinz Real Chair Professor of Business at NYU Stern, where he holds joint appointments in technology, operations and statistics, TOPS and marketing departments. He also serves as the Director of the Masters of Business Analytics and AI at NYU Stern.
Beyond his academic work, he has advised and consulted major companies his research has generated profound insights and shaped how organizations think about the economic impact of platforms data and AI driven transformations. He’s also wrote a couple of seminal books one Tap – unlocking the mobile economy and most recently is the co-author of Thrive – maximizing well-being in the age of AI, which looks into how AI can enhance human potential and especially business strategy, which is our biggest topic on this podcast. So Anindya, first of all, I would like to give you some space, let’s say, for you to allow our listeners to catch up with your extensive experience in the space. And also, as we were discussing a little bit before, situating, you know, your work in the moment we live in AI, which is definitely a defining moment, pivotal moment of the technology and society, most likely. And also, of course, you have a book that you just recently released. So it would be nice to hear from you how you got into the thesis in the book. And I think this can be a good basis for conversation for today.
Anindya Ghose
Okay, that sounds great, Simone. Thanks again. So maybe I’ll start by saying that I’ve been, I guess, immersed in that AI space for almost 25 years in like three different capacities, research, consulting, and teaching. And I guess it’s fair to say that we were working on AI before AI became cool. We were the geeky nerdy kids at that time. And most of the world would dismiss AI at that time as something very niche and not very relevant.
I guess, chatGPT changed everything – we finally, maybe you can call it the cool kids, something about the lines.
So what I have done in my work over the last couple of decades is even in my research and definitely in consulting, I do a lot of hands-on work helping companies and organizations figure out what to do with their data. And so typically the conversation starts in a very simple way. And it starts by asking – people are telling me that, we have a lot of data and we don’t know quite what to do with it. And so can you help us figure out what to do with this data set? And that’s how the conversation typically starts.
And if you go back to the last maybe 15-odd years, we went through these waves. We had the big data wave first, then we had the business analytics wave, we had the data science wave, and now we have the AI wave. And the thing that’s common across all of these waves is the power of using data-driven decision-making. And what has basically been changing over the last few years is the infrastructure, the fundamental infrastructure that organizations can leverage to use this data. And so we have now come to a situation where I think most organizations can take the data. They have access to the infrastructure as well and they can actually do a lot of meaningful stuff with it. And the only difference is, you know, what is the right way to proceed? You know, it’s become less of a should we proceed, it’s become more of a what is the right way to proceed. And so the final thing I’ll say here is the book that is written, co-authored with another colleague of mine, Ravi Bapna, but he’s also spent a lot of time in the industry helping companies.
And what we thought we would do is we will help organizations and corporations and companies put together a framework, which we call the House of AI framework, that will take them from basically zero to 100 in this AI transformation journey. And I’m happy to elaborate what this House of AI framework is.
Simone Cicero
Okay, I mean, that’s great. I would love actually for you to dive deep into the House of AI framework because I believe that is gonna give us a bit more, I would say an understanding of your particular stance on AI, which when I looked up with your work and the book, it sounded to me very pragmatic, something that we normally lack a lot in the discussion around the organizational adoption of AI.
We lack pragmatism, we lack business alignment. Everybody talks about it, but very few, I would say, obtain real business outcomes from it. So I would love if you can expand from this perspective, right? So how can we get real about AI, even beyond all the buzzwords and the excitement around Gen.ai. How do we get real about AI?
Anindya Ghose
Yeah, I’ll keep it very real because that’s basically what I do. So the first layer that when I helped organization, and I should say that just to give some idea, I’ve worked with almost 60 companies now in all five continents, in a multiple industries.
The first layer is what we call the data engineering layer. Here, what we are doing is we are collecting all of the data that these companies have. And we are essentially saying: “look, all of the raw data you have is very messy. It has a lot of problems. There are missing variables, there are missing observations. It’s not formatted well for any queries. So we are going to be using some big data tools like SQL or Hadoop or even Python to clean this messy data and fix all these problems so that it can be curated and formatted well for the next step.”
And before I go to the next step, I’ll say this. Most organizations I’ve seen over the last several years make the mistake of not spending enough time in the data engineering layer, because it’s not a very exciting part of this AI journey. The exciting part is what comes next. The exciting part is the next layer consists of four different questions based on what you’re trying to achieve. So the first objective is, the manager, the CEO, the CMO, CIO, et cetera, saying, okay, tell me what has happened so far. That’s a very descriptive question. Tell me what has happened so far. I don’t want to do anything else yet. The second question is what we call the prescriptive pillar. Tell me what will happen next. That’s a predictive pillar. The third question is what we call the causal pillar. Tell me why did something happen? And the fourth question is the prescriptive pillar, which is, tell me what should we do next?
So the four pillars are – what has happened so far? What will happen next? Why did something happen? And then what should we do?
And for each of these four pillars, we are basically helping companies build the appropriate machine learning or statistical model. So the first prescriptive pillar is basically what we call – think of like data visualization. You are essentially fishing to figure out what has happened. The second is predictive. Here we are doing data mining, forecasting, machine learning. The third is causal inference, which is an incredibly difficult but a very important pillar. And here we are helping companies run experiments. We are teaching them econometrics and statistics. And finally, there is the prescriptive pillar, where we are helping companies do decision modelling under optimization.
So as you can see, these four pillars are very dependent on the data engineering layer. And it’s very cool to work on these four pillars, but it’s not very exciting to work on the data engineering layer. But unless you clean the data, you are basically left with garbage in garbage out. And the biggest mistake companies make is instead of spending more of their time cleaning the data so that they can prevent the garbage in garbage out, they jump right into model building.
And my rule of thumb is you should spend at least 70 % of your resources on data engineering and the remaining time on these model building. The last thing I’ll say is on top of these comes GenAI. So GenAI, as you know, is relatively new. It’s about two and a half, three years old. Before GenAI, we didn’t have access to some of these LLMs and some of these pretty exciting tools.
But the last layer is what I call the storytelling layer in a way that is fair and ethical and equitable too. Okay, so as we know, the book that Ravi and I wrote talks a lot about the positive effect of AI on business and organization society, but that is not dismissing some of the negatives that has also happened. And one of the problems that AI has is it can be, if it’s not de-biased, it can be unfair, it can be unethical. And so,
The last layer is when we say, have you checked everything so that when you’re finally telling the story and taking the recommendations, you are actually debiasing and making it fair and it’s making it transparent, okay? So those are the three steps.
Simone Cicero
I mean, I know Shruthi if you have any other questions coming up, I wanted to just maybe underline one thing before we move on into other topics. Is that you seem to be, and also I quote today’s when I was listening to some of other conversations you had, you seem to be very – to advise companies to really develop a certain intentionality on when they adopt AI, right? And this is somehow resonating with some of the ideas that we also are getting to when we talk about, think about AI. So it looks like that if you don’t as an organization, if you get into AI just, you know, with this posture of churning, sorry, ingesting large quantity of data and just see what you can get out of that without any modeling of your business process, modeling of your organizational context, your strategy, your, for example, ethical constraints or your vision as a company – It can be really dangerous because you can really end up in not being able to observe what this model actually do and really you end up in a situation where you can really get much strategically speaking out of your adoption of AI.
Am I right? So am I right to that? The intentionality, all the prior, because when you say, for example, cleaning up the data, for me, it talks a lot about understanding your data ontology, for example, understanding what’s happening in your business, what you want to achieve, what your customers want. What is your model of the system you run as an organization. Is it very important in your experience to build a representation of the system you are in to really reap the benefits of AI and GenAI more specifically?
Anindya Ghose
Yes, I would say in a nutshell, yes, but I also explained that. So what I mean, what we mean in the top one book is that intentionality that you talked about, Simone, is very much relevant from day zero. Okay, so from day zero, you’re looking at the data generation process to understand why, you know, a certain segment of data is coming out in a certain way. Okay.
Sometimes the data has anomalies, sometimes they have outliers and anomalies and outliers on their own are not problematic. You may have certain customers or certain suppliers in your organization that are outliers and they are generating outlier data. So it’s not a data problem, it’s just an actual real world problem. So the fix to that is you’re not cleaning up the data and removing the outliers because these are actual outlier behaviors demonstrated by suppliers and customers. That’s what the intention really comes in.
So you’re right. I think… And the last thing I’ll say is the reason this intention is important is if you go all the way without being intentional about how you build this infrastructure and how you adopt AI, then you have to go back and course correct. That’s a very costly process because you already invested in the infrastructure. So rather than that, from day zero, do very methodical planning about what you need to do to get to a certain stage so that you don’t have to do this major course correction later on.
Shruthi Prakash
Essentially, you also sort of touched upon this point of focusing more about the positives, right, in your conversation. one, I would like to touch on that, let’s say focusing on AI’s benefit in terms of, let’s say, I think you mentioned on health, environment, and so on, versus focusing on, let’s say, the biases danger. So, how do you essentially first change the language and communication around that and sort of deeply integrated into an organization and see AI as a problem solving tool rather than how it is today as just an automation tool essentially.
How do you operationalize it? How do you change the language and conversation in organizations?
Anindya Ghose
That’s a really great question and I have so many thoughts in my mind I have to figure out how to say this crispy. I’ll say this. There are three kinds of people I met in the world. When they hear the word AI, one kind thinks about automation, second kind thinks about innovation and third kind thinks about regulation. Okay. Now there is a role of AI in all three. The question is like in what order do we go? And the second conversation Shruthi on this is – when I see two different kinds of people, when I see AI, they see safety, another kind, when they see AI, they see opportunity. So who are you? And so I think, like, when I start organizational conversations, I typically pose one of these two questions. When you hear the word AI, like, I often do an informal poll with the board or with the CSO and say, OK, when you hear the word AI and you have two choices, you hear safety or opportunity, where do you and not surprisingly, there is a split. Some people go with AI’s opportunity. So it’s very positive, right? And other side is AI safety. So yeah, we have to be cautious. It’s risky. It’s more on the negative side. I’d start with there. And then the thing that I always said at the beginning is that both Ravi and I wrote this book not to dismiss the negatives. We are cognizant of the negatives.
It’s so down that until we wrote the book, know, all the storytelling about AI was very negative, like fear mongering. was all about, can we talk about the risks and the safety and the caution? But there was that there was a lack of this, you know, positivism that is also applicable to AI. And so the book we wrote is about not dismissing the negatives because there’s already a cottage industry that is throwing out, you know, the panic and the fear about AI taking our jobs away and all that.
But we said, let’s also look at all the positives. I mean, you know, like mental health, physical health, relationships, work, career, education, financial literacy. We document in every aspect of human lives, we document how AI has improved things. So I think that was part of the reason, you we wanted to kind of bring the narrative on the other side and say, let’s also talk about the positives.
Shruthi Prakash
So I was just curious in this that for me, this has always been a personal, I guess, challenge that is AI therefore developing a little too fast in comparison to, let’s say, its impact. And I say this because you mentioned the point on mental health as well, right? Like there have been so many cases where the speed of development has been a deterrent in its path. So one, is it therefore – too fast in its development and therefore, how does let us say the governance and things like that seep in.
Anindya Ghose
Yeah, it’s another very great question. mean, I would say, you know, my own, I’ve been myself surprised by how fast this is happening because look, like, you know, like I mentioned before, I spent like 25 years actually building these algorithms, coding, you know, these models and all that. And, you know, those days we would make good progress, but nothing as rapid as what we are seeing now. Okay.
And I think the fundamental trigger has really been generative AI. I think large language models have just fundamentally made this curve instead of a linear curve, it has made an exponential curve. There’s no question. And I think I can totally understand why some people will feel pressure or even have some mental worries about what is this all going to mean for me, my family, my children, and so on. I don’t think I have all the answers.
All we can say is we have some perspective on how this might unfold. But I also like to your point, I’m not dismissing of the fact that this is moving fast. And on one hand, it excites me. Okay. It excites me that, wow, there’s so many things we can do and we are so much more productive, you know, like I, I mean, I’m just one single individual, but I can definitely tell you I’ve been a lot more productive in the last two years than before. Thanks to LLMs.
But I do understand why people have to maybe take a step back sometimes and think about, okay, are we doing it the right way?
And so that sort of brings us to questions about leadership, culture, organization, design, and so on.
Simone Cicero
I’m wondering, especially as we, if I look at the framework that you shared when we started, that goes through descriptive, predictive, causality, and prescriptiveness, it looks like that now with agents, for example, we are moving into a new stage where you can actually move out of intelligence into AI as a – you said a multiplier of productivity, but we are increasingly actually seeing AI as agents. I think the idea of an agent goes well beyond productivity. It goes into really a new regime for organization. It looks like an organization that can leverage on AI agents. It’s really different from an organization where you have sent out so people that actually use AI to improve their productivity.
Because suddenly when you move into AI agents, you have to oversee the work of the agents in a much different way. You don’t have this embedded humanity inside the work. It’s more of work that you have to control because it can become radically disruptive. If it hallucinates or it goes out of control, know, controls and the red tape.
So what is your experience in starting, I guess, to help organizations move into this new space where AI can actually be a force of production for the organization, not just an element of intelligence? I’m also interested to ask you, because I know that your most recent work, it’s also about how to adopt this technology in a fair and ethical way.
And to some extent, I believe that these technologies are now obliging organizations to ask questions in terms of, again, fairness, their ethical values. So how is this happening? As we move into AI as a productive extension of the organization, maybe imagining scenarios like a business unit completely run by AI agents – what does it mean for the organization? What are the things that you are encountering in your pioneering work?
Anindya Ghose
Yeah, so it’s a fascinating question, Simone. I think rather than thinking of AI as an intelligence amplifier for decision-makers, suppose we start to think of AI as a force of production. AI is a tool that directly reshapes how work gets done. So I think we take this perspective, and then AI is not just about refining executive choices, but fundamentally altering workflows, automating tasks and potentially redefining the structure of firms. So I’ll talk about maybe three things at least based on what I’ve seen. I would say at the outset, should say that this is still very, these conversations are happening. Like I’m on the board of several companies, we are still in conversation mode, just so you know. I am yet to see an actual implementation of an AI agent, but I think it’s good that at least this conversation is happening and the kind of questions you’re asking are exactly what the boards are asking or what the C-suite is asking the board or vice versa, right? So what I tell my fellow colleagues in company boards or the C-suite is I can think of three things. When AI is viewed as a force of production, it becomes a transformative tool. For example, automation of routine tasks, okay? AI agents can take over repetitive rule-based tasks, which will free up human workers for more creative or strategic roles.
Okay, number one. Number two, I can think of AI as an augmentation of human labor, where AI is actually enhancing human productivity by providing real-time insights, predictive analytics, like the House of AI framework, even sometimes co-piloting complex tasks, which I call AI-assisted design, AI-assisted coding. So that’s an augmentation of human labor. And the third is creation of new workflows. So AI can enable new ways of working, such as Generative AI is now creating content for media agencies and ad agencies, or even AI-driven supply optimization.
So the common theme across all three, automation, routine tasks, augmentation of human labor, and creation of workflows is AI is not just a tool for better decision-making at the top. It’s a fundamental driver of how value is being created in the organization because we are seeing AI as a force of production and not just an intelligence amplifier. Okay, and this has many implications for organization design too, which you know we can talk
Simone Cicero
Yeah, I think this is a good segway. I have a bias for these type of conversations because in general, think at Boundaryless we have been pioneers in understanding that as organizations have evolved towards more complex systems, You fail if you think that you can manage them with a managerial perspective, with a bureaucratic perspective that comes out of the 20th century.
But rather, what we advise companies to do very much originally was to move into an architectural leadership. So we believe that companies need to set the constraints for the employees to thrive and to be very much autonomous like an agent would be. Agents would be very autonomous in doing work. And it’s funny sometimes that when we think about employees versus agents – we tend to put more agency in an agent, in an AI agent than in an employee, which is super funny, you know, if you think about it. Actually a bit not that much funny if you think about the human implications. But let’s say that first of all, we have to move into an architectural approach to organizing, you know, to the organization so that you can create these spaces where maybe today employees tomorrow agents can explore and find their own opportunities and execute the work.
And on the other side, another thing that we seem to see as a recurring critical point in this evolution is for organizations to, as we said at the start, really build a systemic representation of what they do. So building their own, discovering their own ontology of work, their workflows, clarifying them, visualizing them, really be able to see what happens here, what happens there, how the pieces connect. That’s very important for modern organizations to be thoughtful and conscious of the work they do.
So this is certainly one piece. But the other piece I think that I’m also curious to hear from you about is suddenly, if you move into this approach of an organization which is more distributed, where you rather create the fences for work to happen, more than dictating what people should be doing. It’s really a post-managerial perspective. It’s really a perspective where traditional management functions don’t have much role to play anymore. So what is your experience? How disruptive is this conversation around adoption of AI becoming from the perspective of management? How is it changing the culture of management in the organization?
Anindya Ghose
Yeah, so I think it’s a fascinating set of questions. And I would start maybe by saying that if you think about the implications of AI as a production force for organization design, the first thing I’ll start by looking at is, there a possibility of displacement of the managerial role? And I think yes. For example, traditional managerial role involves information processing, right? I think that becomes less critical now because AI is being able to automate information processing.
in the house of AI framework. The second layer is all about information processing. So this could then shift, this could lead to a shift from hierarchical management structures to more flatter, more networked and more autonomous teams, right? And then if you think about, mentioned post managerial, I think I agree. I mean, I think for example, you know, if AI is handling the coordination and optimization, then organizations, some organization I should say, might move towards more algorithmic management where decision-making is distributed across AI-driven systems as opposed to like concentrated at the top. So like, think, mean, my experience is like when I see Uber and Amazon, they’re already using AI to manage logistics and workflows in a way that reduces the need for middle management.
If you see the structure of Uber and Amazon, the middle man limit is not as thick as it used to be. And the third thing I’ll say is, look, I think when it comes to decentralization and distributed decision-making, AI is enabling these data-driven decision-making across all levels. So I almost feel, and this is a hypothesis, it’s not emergent. I almost feel this DAO-like structure, decentralized autonomous organizations where smart contracts and AI agents and decentralized governance, I almost feel like we will probably see some version of that, not in the entire organization, but in part of that organization. And if they see that that DAO-like structure is becoming successful, I think then we will see more expansion.
Now, in the middle of all this, I think I missed answering one question you asked before, which is, where does fairness and ethics and equity and all – And I think that’s an incredibly important thing because if I’m going to put more agency on an AI agent or at least equal agency on agents and human beings, I have to make sure that the data on which the agent has been trained has been de-biased. And the good news is de-biasing is a lot easier than we think it is. I mean, yes, we need some data scientists to do it, but you know, like as an educator, I know it can be done. We train our people in doing it. So the only question is, is the C-suite, are they willing to spend resources, valuable time and money to de-bias the data so that the AI agent actually behaves in an unbiased and fair manner? OK, so that’s why that data engineering layer, you have to de-bias the data in that layer. And otherwise, that agent is trained on biased data, partial unfair not unfair, very partial data. then the agent is not going to be able to generate its own intelligence, because intelligence is based on pre-training and fine-tuning. You have to fix that data at the very beginning and then let the agent train on unbiased, debiased, fair data. Then the agent is more likely to be equitable and fair.
So I think if we have to move towards this kind of new organization structure where agents have some agency, we have to make sure that CEOs and the CIOs, CTOs especially, are willing to spend time and resources in de-biasing the data at the very beginning.
Shruthi Prakash
Sure, so I mean, for me, it sounds a lot like ecosystems now need to be developed in an AI centered sort of fashion rather than, let’s say, human-centered fashion. For me, I’m curious, does that like decide there in the kind of outcomes we can expect from an organization? How do we, let’s say, top leadership or senior leadership in order to prepare for an AI dominated sort of organization?
And lastly, who is to succeed in all of this? Are, let’s say, larger organizations going to have an unfair advantage in this journey over small organizations because the system of concentration of power is essentially shifting. So, how does all of that play out?
Anindya Ghose
Yeah, all the great points, Shruthi. And I would say this, that my personal belief and preference is not for AI to dominate humans, but rather I believe in AI and human complementarity. I almost want AI and humans to have equal roles. And maybe at the beginning, I still want humans to have a more important role than AI. So I’m not saying that organizations should jump into AI agents right away.
It’s also true with that, you know, it’s inevitable. So it’s like you cannot prevent it from happening, but it’s so much more cost efficient. So in my personal belief, an ideal world is where AI and humans actually coexist and there’s complementarity and rather than, know, substitution. Now that being said, the challenges you brought up are a hundred percent valid, right? So like, what am I, so when I have these conversations, you know, here are the kind of challenges that we are often told to think about, right?
So number one, AI will not always make fair, ethical, strategic decisions unless, like I said, you will have spent time and resources on debiasing. That’s what we call loss of human oversight. So the risk of loss of human oversight is that there may be, unless the algorithm has been debiased, some unfair decision being made. Number two, there’s going to be job displacement and social resistance.
Like the question Simone asked before, like the shift to post managerial structures, right? I think I would invite some pushback from industries that are reliant on middle management because that’s where I see most of the cutbacks happening. Not at the bottom, not at the top, at the middle management, right? And so I think that employees and managers will resist changes brought by AI, particularly if it threatens their roles, right?
Then you mentioned about skills, right? I do think there’s a big skill gap. Organization have to invest in upskilling their workforce to work effectively with AI. And maybe that’s why I say we, as an educator, we have launched all these specialized master’s degree programs in AI. And a lot of our students are actually senior executives. So I think that’s a good news that they recognize the importance of upskilling. So they come back to the school and classroom and do it themselves.
And the last thing I’ll say that if we actually end up in a world where there is fewer traditional management and middle management, okay, who is going to be responsible if and when AI driven decisions go wrong, right? Like that’s to me, I don’t have an answer, but I do see that problem occurring. Who’s responsible in the middle management when AI driven decisions go wrong, because you know, they will go wrong. They are also learning.
So I think these are fascinating questions. We don’t have all the answers, but this is important to ask these questions.
Simone Cicero
I mean, this conversation is bringing me to a very interesting place, I think. I’m getting into some really interesting questions that I wanted to share with you, some considerations at least. So if I look at what you said,
Yeah, because essentially when you say, when you introduce it should be this topic around AI design and systems, right? So essentially envisioning this perspective where AIs have much more, much bigger footprint in designing and taking choices, let’s say, in calling shots in regards to what we design, what the organization does and so on. Anindya, you said first, before you said, we’re potentially moving into algorithmic management where I can imagine an organization has a little bit of an architecture overall, a model, or at least a series of models that interact with these architectures, smart contracts that regulate the relationship within these units that are bringing forward to the production and possibly I was thinking, how do you program ethics into these? It looks like ethics needs to be programmed, first of all, into the models, the LLMs. You spoke about debiasing and so on. And maybe on the other hand, you can embed some ethics or in general, some culture, organizational culture in maybe the contracts that you allow the nodes in the organization to, let’s say, discuss, agree, and create between each other.
So for example, you can have an organization where you allow units to share, for example, keep a lot of their revenues or versus an organization where it’s more like a systemic, general performance that you measure as a whole. So you have these cultural choices to make that can be embedded in the contracts and in the technologies that the organization uses.
Suddenly, I was thinking about something that I shared a few weeks ago, a few months ago on LinkedIn. was thinking that if we envision a future where AI is more prominent in dictating choices, for example, right? So they push more into suggesting what’s good or what’s wrong, what’s right or what’s wrong. They may end up with a lot of common sense that end up in not being applicable because of the existing lock-ins in society that are pretty human, not, let’s say, not AI generated. They are pretty human, meaning that, for example, lock-ins in capital, lock-in in positional rents, or things like that.
So the question I wanted to ask you is, if we really look into a perspective where AI takes more important role in deciding what we build, how we build it, how we bring it into the market, and so on, what happens with differentiation or competition? Can we look into a market where the common sense, the open interfaces, the collaboration becomes more of a standard, more of a first choice versus maybe you know, competition for the sake of optimizing, you know, certain positional rents or making a difference, a competitive difference between each other.
So are will looking into a more open, more collaborative market where common sense is more of a substantial element in the conversation.
Anindya Ghose
Yeah, think, you know, there’s obviously like a lot of issues here to kind of maybe unravel, right? The first thing maybe we’ll start with like the ethics question you asked, which is right. So I think there’s an important nuance between, you know, like ethics and fairness. Okay. And so what do I mean by that? Right. So I think the fairness problem that AI can create can be solved by de-biasing and training it on the right data set. Like a data set that is more generalized. It’s not a sub-sample of the population, right? It’s a representative of the population. So like to me, as someone who’s like build these models, right? I know that we can solve the fairness problem. The ethics issue comes, you know after the AI has given recommendations, a human often has to make the decision. And that’s a bigger problem because it’s not easy and it’s very difficult to teach people ethics. I just sometimes feel like ethics is, you either have it or you don’t have it, okay? And so how do organizations deal with this? I think right now the issue is about incentives and penalties, okay? So not surprisingly, if you are caught being unethical in some implementation of AI, then you will face severe penalties. It could be job loss, could be demotion, or whatever.
And if you are seen consistently performing in an ethical way, you are incentivized with some something, right? So I would say that, and I wanted to like devel in the different between fairness and ethics because fairness is a problem that is coming out of AI that we can fix. And ethics is a more of a human thing because it comes at a stage where the human being has to implement the output of the algorithm. And I may have my own preconceived notions. And if I’m not incentivized or penalized, I might not behave in ethically.
Simone Cicero
Yeah, but I don’t know if I interrupted you, but I take the chance to really clarify what I was saying because I feel it wasn’t totally clear. What I mean is, for example, when you say it’s hard to teach people ethics, I guess that it’s paradoxically maybe easier to teach it to an AI. Am I right?
Anindya Ghose
Yeah. Okay, yes, yes, because up until now, the AI is not necessarily generating its own intelligence. We will be in a world soon when the agents will generate their own intelligence, just like human beings do. At that time, I don’t know what will happen. At the moment, the agents are acting 100 % based on the training that we have given them.
And so if you have trained them right, the agents will behave in the ethical way. Because human beings, in our mind, we can change, we can deviate. But the AI agent will not deviate yet. But Simone, if two years from now I do see a world, and not just me, like people much more smarter than me have said, in two years from now, in agentic world, these agents can generate their own intelligence and make decisions independent of the data that they have been trained on. In that world, it’s very hard to predict what will happen. We have to wait to see. I think that’s an open question.
Simone Cicero
I mean, that’s very interesting because I think it leads me to maybe the last question that I would or the last topic that I would like to bring on the table for today before we move into the breadcrumbs in the closing, which is what do you see happening with competition then? Because of course, know, a lot of the differentiations that companies have at the moment on the market is due to their culture, their particular vision capabilities that are unique, right?
Of course, know, some competition advantage is due to maybe your assets, like infrastructure or things like that. But let’s say that you have two or three or four of all hundred companies that are on par in terms of assets, capital, infrastructure. How is it going to hand? know, a lot of AI is based on the same model that we know do not generate any particular competitive advantage the architectures are becoming very common.
So what we see for example, from our observatory, we see that there is a common protocol of organizing that is emerging, know, micro enterprises, shared services and so on. technology is there, it’s common for everybody. So how’s gonna be, how can organizations really make a difference and compete with the others in a landscape where these technologies level.
Anindya Ghose
Yeah, that’s a really great question, Simone. I have a one word answer I’ll explain. It’s people. Okay. So what do you by people? You’re absolutely right that AI is now not on its own. It’s not becoming a differentiating tool. If everybody adopts it, and you’d have seen this going back many, many decades of technologies, any general purpose technology that gets disseminated in society and is adopted by every company, that differentiation goes away over time because now it becomes a commodity.
Right? I think, and what I believe is happening, at least based on all these companies that I’ve actually helped with hands on is the differences in the people, okay? People’s skills, people’s intentions, people’s mindsets, people’s thinking. So what I mean by that is, all these companies, like, yes, they have access to the same LLM, the same cloud computing, the same data engineering, the same descriptive prescriptive models, because we’re telling them to do so.
But there are differences in the kinds of people they continue to hire and empower. So there’s two things that I can think of. One is there are differences in the skill sets that people are having across organizations of the same structure, and same capability. And there are differences in leadership and empowerment. And so I think the winners are the ones who are basically thinking about empowering more on the data, let’s say you have the data science team, empower them more to make decisions rather than the middle management continuing to make those decisions. So, like we talked about, I think AI is going to reduce the need for middle management. Leadership is going to get more distributed. We’re going to have more adaptive network leadership. So the organization that make these changes faster will have the differentiating advantage.
Because there’s not going to be much of a differentiation on the technology on the toolkit. They are about the same. Everybody will have it.
Shruthi Prakash
If I can just add to that, right? mean, for me, I’m curious, like, is it going to be sort of like a winner takes all market in the sense that whoever integrates AI better has, let’s say, exponential advantage in that sense? Because even though the platforms might be the same and the access to the platforms might be the same, but let’s say the amount of volume of data, the better precision of data, the awareness of a consumer, et cetera, all of that is totally different. Right.
So is it going to be that case where once again, you know, it sort of goes in that winner takes all sort of direction.
Anindya Ghose
I think so. don’t know about winner take all, but I think the winners will stand out and there’ll be the first more advantage. I often think like the very first time I started working in big data was in 2009, almost 16 years ago. And I came across a company that we all know, a company called Procter & Gamble. It was a fascinating experience because in their Cincinnati office, P&G, the headquarters in Ohio in the US, they have this room called the cockpit room – which is a room full of massive computer terminals. All the walls are full of computer terminals.
And the senior management meets frequently in that room. They spend the full day in that room, know, once a month or once every two weeks, just to make data-driven decisions. Okay, that’s all they were looking at, looking at dashboards and pie charts and flow charts and all that. This is 2009, right? And so PNG has continued to be a pioneer in adopting these, you know, forward-looking technologies.
And that’s why it had done so much better than many other consumer-packaged good companies. And I think like similar things, you’ll hear stories in like hotel chains, airlines, and et cetera. I do think that we’ll see similar stories unfold where in each industry, there’ll be one or two leaders. I wouldn’t say winners, but leaders. And those leaders will always be the shining beacon. And any case studies will be written about them, and so on. So I do see that.
Simone Cicero
Thank you so much. I I’d love to continue this conversation, but I’m conscious of the time we have. So I would love to ask you to share as we close – couple of breadcrumbs for our listeners to catch up with, especially based on your wide experience and your capability to really integrate further than technology.
We’d love to hear what you suggest to our listeners to explore and catch up with in terms of resources.
Anindya Ghose
Sure, I mean, as an educator, I’m probably a little biased, but I do believe that one way of catching up or even getting started is to get you or your people in the organization trained, right? Upskilled and trained. And I think figuring out the right university and the right program and the right degree to tell and send your people to upskill is very important. And it’s not something you can learn entirely on your own.
So I think I would encourage companies to think about sending people or the executives on short one week, three day, six week programs to get up skilled. The second thing I think is, essentially I’ll tell leaders and organizations to just like every other general purpose technology, this thing has the good, the bad, the ugly. And I think.
Why is it important to think of AI as cautious and safety? Think about it as an opportunity. Think about innovation as opposed to maybe automation or regulation. And the third thing I’ll say is outside of the organization setup is there’s some fascinating things. There’s an AI war being fought between the US and China. So keep an eye out. I don’t believe in this let’s win the AI war because many of my colleagues here or even the regulators and politicians I often have to work with, they will say, we have to win the AI war. And I don’t really know what that means. We don’t need to win the AI war. We can coexist with another country that is also very, very good. I do think there’s a place for both the US and China to have a very important role. And I don’t think we have to win the AI war. So I think we have to coexist.
Simone Cicero
Thank you so much. I hope you also enjoyed the conversation as much as I, as we did.
Anindya Ghose
Yes I did, I loved it. Thank you Simone and Shruthi, it was awesome. Loved it.
Simone Cicero
I hope we brought you to some uncharted territories as it seems, and we are all sharing this curiosity for what the future will bring. So thank you so much for being available for these conversations. And Shruthi, thank you for your questions as always.
Shruthi Prakash
Thank you, thanks Anindya, thanks Simone.
Anindya Ghose
Thank you, thank you both, yeah. This was fun.
Simone Cicero
And yeah, for our listeners, as always, don’t forget that you can head to the website, boundaryless.io/resources/podcast. You will see the episode with all the notes and the topics that Anindo spoke about, some links and references. And until we speak again, of course, remember to think Boundaryless.