Making AI-ready Organizations – with Thomas H. Davenport and Laks Srinivasan
BOUNDARYLESS CONVERSATIONS PODCAST - SEASON 4 EP #8
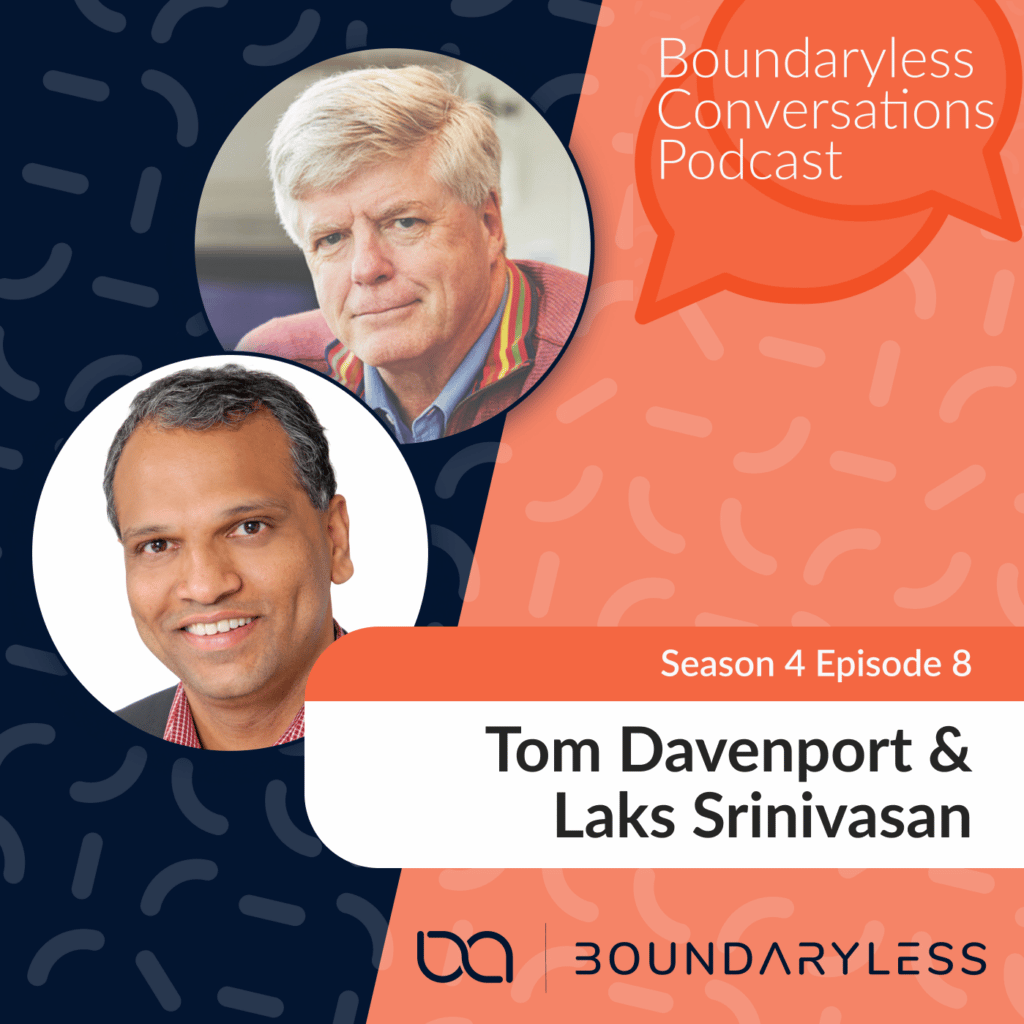
Making AI-ready Organizations – with Thomas H. Davenport and Laks Srinivasan
In this episode, we talked to Tom Davenport and Laks Srinivasan from Return on AI Institute (ROAI) about how AI is empowering and challenging organizational models worldwide.
Podcast Notes
Thomas H. Davenport is a world-renowned thought leader and author on AI. He is the President’s Distinguished Professor of Information Technology and Management at Babson College.
Laks Srinivasan is a data and analytics executive and he is a co-founder and Managing Director at ROAI and former CEO of Opera Solutions (now ElectrifAI).
Tom and Laks explore with us how different forms of artificial intelligence might transform product teams at companies around the globe. In the second part of this episode, Tom and Laks offer concrete examples of companies that have created new business models powered by AI, as well as suggestions on what traditional organizations should look at when preparing to adopt artificial intelligence.
At Boundaryless we’re partnering with ROAI to explore the convergence between AI and Platforms, check out our research and services here: https://blss.io/ROAI
Key highlights
- AI is becoming pervasive in large organizations, but many are still struggling to get meaningful value out of it.
- Companies that “do AI” vs (digital native) “AI companies”
- Platform business models (as a form of ecosystem) are based on AI
- How AI could transform product teams
- The challenge with AI is multi-dimensional: involves organization, leadership, culture, data and technology.
- AI replaces tasks rather than entire jobs.
- Strategy-by-doing applies to AI: think big, start small, fail fast, and invest where things are working.
- Increased awareness among executives is needed to develop their intuition around AI.
Topics (chapters):
(00:00) Intro notes and welcoming of Thomas H. Davenport and Laks Srinivasan
(03:16) How AI is empowering organizations or challenging organizational models.
(08:11) AI as a matter of doctrine in organizations: yes or no?
(11:56) Platform business model (as a form of ecosystem) based on AI
(17:13) How AI could transform product teams
(24:50) Example of companies which have created new business models powered by AI
(33:40) What should traditional organizations look at when preparing to adopt AI?
(42:02) To integrate more AI into the process? Think big but start small.
(49:58) Thomas and Laks’ breadcrumbs
To find out more about Tom Davenport’s work
- Twitter: https://twitter.com/tdav
- LinkedIn: https://www.linkedin.com/in/davenporttom
- Website: https://www.tomdavenport.com
To find out more about Laks Srinivasan’s work:
- Twitter: https://twitter.com/LaksSrinivasan
- LinkedIn: https://www.linkedin.com/in/laks-srinivasan
- Website: https://roaiinstitute.com
Other references and mentions:
- Working with AI https://mitpress.mit.edu/9780262047241/working-with-ai
- All-in On AI: How Smart Companies Win Big with Artificial Intelligence https://www.amazon.com/All-AI-Companies-Artificial-Intelligence-ebook/dp/B09Q6KHWVT
- Ping An Group https://group.pingan.com
- CCC Intelligent Solutions https://cccis.com
- USAA https://www.usaa.com
- Amara’s Law https://en.wikipedia.org/wiki/Roy_Amara#Amara’s_law
- The Return on AI Playbook Podcast https://sites.libsyn.com/411860
Tom and Laks’ suggested breadcrumbs (things listeners should check out):
- Being a volunteer for fire control or, in general, being a volunteer for something
- How Generative AI Is Changing Creative Work https://hbr.org/2022/11/how-generative-ai-is-changing-creative-work
Recorded on 28 October 2022.
Get in touch with Boundaryless:
Find out more about the show and the research at Boundaryless at https://boundaryless.io/resources/podcast
- Twitter: https://twitter.com/boundaryless_
- Website: https://boundaryless.io/contacts
- LinkedIn: https://www.linkedin.com/company/boundaryless-pdt-3eo
Music
Music from Liosound / Walter Mobilio. Find his portfolio here: https://blss.io/Podcast-Music
Thomas H. Davenport is a world-renowned thought leader and author on AI. He is the President’s Distinguished Professor of Information Technology and Management at Babson College.
Laks Srinivasan is a data and analytics executive and he is a co-founder and Managing Director at ROAI and former CEO of Opera Solutions (now ElectrifAI).
Tom and Laks explore with us how different forms of artificial intelligence might transform product teams at companies around the globe. In the second part of this episode, Tom and Laks offer concrete examples of companies that have created new business models powered by AI, as well as suggestions on what traditional organizations should look at when preparing to adopt artificial intelligence.
At Boundaryless we’re partnering with ROAI to explore the convergence between AI and Platforms, check out our research and services here: https://blss.io/ROAI
Key highlights
- AI is becoming pervasive in large organizations, but many are still struggling to get meaningful value out of it.
- Companies that “do AI” vs (digital native) “AI companies”
- Platform business models (as a form of ecosystem) are based on AI
- How AI could transform product teams
- The challenge with AI is multi-dimensional: involves organization, leadership, culture, data and technology.
- AI replaces tasks rather than entire jobs.
- Strategy-by-doing applies to AI: think big, start small, fail fast, and invest where things are working.
- Increased awareness among executives is needed to develop their intuition around AI.
Topics (chapters):
(00:00) Intro notes and welcoming of Thomas H. Davenport and Laks Srinivasan
(03:16) How AI is empowering organizations or challenging organizational models.
(08:11) AI as a matter of doctrine in organizations: yes or no?
(11:56) Platform business model (as a form of ecosystem) based on AI
(17:13) How AI could transform product teams
(24:50) Example of companies which have created new business models powered by AI
(33:40) What should traditional organizations look at when preparing to adopt AI?
(42:02) To integrate more AI into the process? Think big but start small.
(49:58) Thomas and Laks’ breadcrumbs
To find out more about Tom Davenport’s work
- Twitter: https://twitter.com/tdav
- LinkedIn: https://www.linkedin.com/in/davenporttom
- Website: https://www.tomdavenport.com
To find out more about Laks Srinivasan’s work:
- Twitter: https://twitter.com/LaksSrinivasan
- LinkedIn: https://www.linkedin.com/in/laks-srinivasan
- Website: https://roaiinstitute.com
Other references and mentions:
- Working with AI https://mitpress.mit.edu/9780262047241/working-with-ai
- All-in On AI: How Smart Companies Win Big with Artificial Intelligence https://www.amazon.com/All-AI-Companies-Artificial-Intelligence-ebook/dp/B09Q6KHWVT
- Ping An Group https://group.pingan.com
- CCC Intelligent Solutions https://cccis.com
- USAA https://www.usaa.com
- Amara’s Law https://en.wikipedia.org/wiki/Roy_Amara#Amara’s_law
- The Return on AI Playbook Podcast https://sites.libsyn.com/411860
Tom and Laks’ suggested breadcrumbs (things listeners should check out):
- Being a volunteer for fire control or, in general, being a volunteer for something
- How Generative AI Is Changing Creative Work https://hbr.org/2022/11/how-generative-ai-is-changing-creative-work
Recorded on 28 October 2022.
Get in touch with Boundaryless:
Find out more about the show and the research at Boundaryless at https://boundaryless.io/resources/podcast
- Twitter: https://twitter.com/boundaryless_
- Website: https://boundaryless.io/contacts
- LinkedIn: https://www.linkedin.com/company/boundaryless-pdt-3eo
Music
Music from Liosound / Walter Mobilio. Find his portfolio here: https://blss.io/Podcast-Music
Transcript
Simone Cicero:
Hello everybody. Welcome back to the Boundaryless Conversations podcast. In this podcast, we meet with pioneers, thinkers, doers, entrepreneurs, and we speak about the future of business models, organizations, markets, and society in this rapidly changing world we live. I’m Simone Cicero and today I’m joined by not very usual cohost, my colleague and cofounder at Boundaryless, Luca Ruggeri. Hello, Luca.
Luca Ruggeri:
Hello, nice to be here.
Simone Cicero:
Luca is joining me today exceptionally because of his previous work on AI and his current work at Boundaryless in integrating elements of AI into our work with platforms and ecosystem. Today we are also joined by two champs of AI and you figure out that this is going to be the topic of the conversation. And whom we asked for some help in navigating the impact that AI is going to have on organizing? One is Tom Davenport, a real luminaire when it comes to AI. Tom has written or edited over 20 books in total and over 300 articles for leading management practice magazines besides teaching at Babson College and Oxford Saïd Business School, among other things. Tom holds a PhD in sociology from Harvard, as well as a BA in sociology from Trinity College. Tom is also the chairman of the Return on AI Institute. Hello Tom, it’s great to have you with us.
Tom Davenport:
Thanks. Very happy to be here and discuss these issues, Simone.
Simone Cicero:
Thank you so much. Together with Tom, we have Laks Srinivasan, a cofounder and managing director at the Return on AI Institute. Laks has more than 15 years of experience in helping clients create real and measurable value from AI as an executive and consultant. Laks holds an MBA from Wharton in Entrepreneurial Management as well as a BS in Electrical Engineering from MIT in India and is currently also an adviser to the European Commission on the DT4REGIONS initiative for AI and big data in the public administration space. Hello Laks, it’s great to have you with us as well.
Laks Srinivasan:
Great to join you guys.
Simone Cicero:
Okay, so let’s jump into the first question. And I would like to ask you a little bit of a recap, I would say on the stages of AI. And now we are seeing so many innovations coming up, and it’s really terrific to see how AI is getting around, even, I would say, in common culture, which is amazing. So what’s coming up in AI? What’s happening? And especially if we look at the organizational aspects in terms of how AI is empowering organization or challenging organizational models. So what are the key trends and maybe something that we may have overlooked?
Tom Davenport:
AI is becoming pretty pervasive in large organizations. I’d say probably over half have something going on. One of the issues that Laks and I have talked a lot about is this one of lack of successful deployment because AI typically involves not just creating a model but also integrating it with existing processes and systems and upskilling people and changing business processes and so on. And data scientists have not typically been that interested in overseeing all those things. They like to create models, but nobody was responsible for all these other activities. So that’s one big activity. I do think that AI is getting much easier to use, and it’s becoming the province of not only professionals, but amateurs in the area of data science. And so you see all these new tools emerging, automated machine learning tools, these large language and image oriented models. I call it generative AI because they create something and they’re very easy to use. And you have artists and writers and bloggers, and probably there will be artificial podcasts before long. I’m not sure there are any yet. But that I think, is really changing the environment. And we saw a little bit of that ease of use earlier just in the incorporation of AI into existing transactional systems. If you’re a sales manager and you’d like to have your salespeople look at the most effective customers to call upon, it’s quite easy now in your CRM system to rank your leads and say which ones are most likely to result in a sale. And the company doesn’t really have to do much in order. I think they have to pay a little bit more to the vendor for the most part to get that AI-based lead prioritization, propensity model, if you will. So pervasiveness, but still challenges in implementation. We’ve been working on that issue. I’ve been working more or less separately from Laks on how people work with AI. I have a new book called Working with AI. I’m co-authored with a professor from Singapore. Then I have another new book coming out soon which talks about really the subject you’d mention some money changes in business models and strategies with AI. It’s called All in on AI, and it’s about companies that really aggressively pursue it, not just for marginal improvement in their operations, but to really change what they do.
Laks Srinivasan:
I think my views are similar to Tom’s. The AI technology continues to advance, as we have seen with generative AI and with text, image, and AI getting into the knowledge worker and creativity space. But vast majority of organizations are still struggling to get meaningful kind of value out of it. So I would say while the technology marches on, the divide continues between the haves and the have nots. And most digital native companies are able to harness the latest advances in technology to be able to get more and more, create more and more value, whereas the vast majority of traditionally organized companies continue to struggle. Though they are all investing as Tom said, 50% of them are investing in AI, but they’re still going after tactical, what Tom calls tactical returns, because they are not yet able to do some of the hard work required, starting with the CEO and the management team, in having that mindset that could create transformational or what we call strategic returns.
Simone Cicero:
So what’s your point of view when it comes to understanding if AI is going to be a matter of doctrine in organizations? Meaning that there is a codified practice and a codified approach is just replicating a doctrine actually kind of a passive series of steps that you have to take in order to integrate AI into your organization? Or if on the other hand, is more like enhancing the responsibility of management and business in general to really understand what’s going on in their business, to really understand where AI as an exceptional capability as these should fit into the picture. So essentially enhancing the responsibility of business to really understand profoundly what’s happening and where AI can be fit into the picture. So would you say it’s more like a general, something like Agile for example, which is more a doctrine element or something more like, I don’t know, design thinking? That is I would say something that requires a deeper understanding of what’s going on to really leverage on the potential.
Laks Srinivasan:
Yeah, I think you’re going to see this theme continue and I may steal some of Tom’s thunder here too, but I’m sure he’s got a lot of thunder. He’s going to come up with some additional insights. But I think it’s both in the sense that for the tactical kind of incremental improvements you do need some methodology. Certain kind of though AI is nonlinear compared to software, traditional software development, you do need a methodology. But for companies to really reach that transformational potential, you do need to have, especially starting at the top, a deeper understanding. Again, we’re not talking about a CEO having to go to a coding hackathon to build deep learning models, but we’re talking about they need to know enough. It’s almost like equivalent of a CFO doesn’t need to be an accountant. The CFO still should be able to understand PNLs and balance sheets, to be able to identify risk, identify opportunities, to be able to ask questions. So I do think for AI to truly make a difference and by the way, AI has been around for such a long time still there’s not that much productivity improvements that we are seeing. Right? So part of that we think is that awareness and understanding at the highest levels in the organization in non-digital-native companies. So you need both to really make this part of how you do business.
Tom Davenport:
Yeah, I think I would agree that you need both. I mean, I always like the analogy that AI is like electricity in that it’s general purpose resource that can be used in a whole variety of ways in business and society. But we’re still if we’re in the early days of electricity and certainly at some point I think you’ll be able to sort of plug and play with AI the same way we can with electricity now. But I think I was reading the other day that ten years after Thomas Edison had identified electric lights still most organizations and cities lack them. So right now, people, executives in particular, really need to understand what these technologies can do, what different technologies are capable of, and how it fits with their businesses. So definitely some in-depth understanding necessary at the moment at least.
Luca Ruggeri:
Very good consideration. I had past experiences also in addressing AI in services for the public administration, so very complex services belonging to institutional players. And the discussion there at that time was about how much competencies do I need to give to the public servants for addressing and for adopting AIs and what was instead the organizational changes needed for correctly use and make a best use of AI services? So my question would be, given everything that you said, what could be the most significant impacts of AI in terms of changing the organizational shape and potential? Given that, of course, it’s not possible not to put everyone in condition to code AI or to be tech savvy on AI, but indeed we can use and we want to use because it’s a powerful enabler. So what changes in terms of organization, in terms of processes, the adoption pattern of AI are needed, or what identification.
Tom Davenport:
I think we’ve seen over the past decade or so, some new business models that really wouldn’t be possible without AI. I’m referring specifically to these kind of multisided platform models that have grown up mostly among digital native companies and are starting to move a little bit into more legacy oriented firms. But connecting buyers and sellers on a large scale really can’t be done effectively without AI. So you can do that sort of matching large scale. A lot of these companies don’t want to have large numbers of employees, so they don’t want to have big call centers. So you need AI to help provide customer service capabilities. I think that’s one major type of organizational change that we already now know. If you look at the Ubers, the Googles, the Airbnbs, and so on, they use a fantastic amount of AI, and arguably their business models wouldn’t be possible without it. And then I know another thing that you guys are very interested in is this whole sort of ecosystem idea, which is, I think, related. But in my recent research about companies that are really aggressive in their use of AI, all in on AI, if you will, I discovered that there are a variety of companies that have these ecosystem business models that also can’t exist without AI. I mean, I think a platform business model is a form of ecosystem. But you take, I don’t know, Ping An, to me, one of the most amazing companies on the planet these days. Five major ecosystems and insurance, banking, healthcare, automotive services, and smart cities, and all of them powered by AI. They’re starting to get sort of relationships between their various ecosystems that are powered by AI. They have a company like Airbus that has formed a skywise ecosystem of all the companies, all the airlines around the world who use Airbus aircraft, combining the data and then doing things like predictive maintenance and route optimization and fuel optimization, so on, again, couldn’t be done without AI. So that to me is the most exciting new sort of organizational model that AI makes possible.
Laks Srinivasan:
I would add to that there’s also organizational changes inside companies as well, if you want to kind of cover that. Tom and I were at a Return on AI summit last week and a chief digital officer of a utility company mentioned that they applied AI in kind of service disruption and recovery or outages due to weather events. And he was talking about before AI they would have a lot of operational folks that would go and maintain things whereas post AI they needed people that have more experience in various kind of nuclear and weather power generation had a lot of kind of certain skills then you need a fewer off them, but very different skill sets. In a way, AI is actually kind of with a human in the loop. AI is starting to make a lot of decisions in an automated way. You just need a few human beings in that particular use case to just do oversight or do exception handling. And that has in some parts of the company profound impact. And then you combine that with what Tom talked about platform models, I mean, we don’t even know what’s going to be possible in terms of how AI could disrupt some of the traditional ways of organizing. And I know you guys are experts in platform.
Simone Cicero:
You know that we speak a lot about how organizations are transforming into empowering more product teams, right? So that product teams can be more independent and autonomous and maybe share some common services inside the organization. And we’re also big proponents of the idea that as teams become more independent and more powerful, also because of some technologies become more decentralized democratized like AI, as they become more powerful, there is a bigger case for teams to be operating across organizations, right? To do more partnerships, more bespoke partnering, I would say, to create a new product. The reaction I was having is given that AI empowers teams, right? So one question could be should we expect that in teams we start to see new roles? Like we have the engineer, we have the, I don’t know, the sales person, we have the marketing capabilities maybe, I don’t know. Then we may think about teams embedding specific AI capabilities and versus how much instead of the organization needs to organize, organize capabilities, I must say functions maybe, or something like supporting platforms or supporting services. So that the organization not only can make the best of AI at the organizational level, so for example, I’m thinking of connecting different products maybe, but also making a case for the employees and the entrepreneurs to build inside the organization versus building on the market as startups, for example, products. So the question is really about how do these two layers, the single team and an org-wide framing of AI, influence the shape that these initiatives should take inside the organization. So if you want also another phase, it could be how can an organization structure itself in a way that can really leverage an org-level org-wide implementation of AI that creates more value than just empowering single teams, product teams with AI functionality, AI capabilities?
Tom Davenport:
I think it’s early to see that with AI. I think we are we’re certainly seeing the emergence of teams to develop and deploy AI. And I think that’s one of the reasons why Laks and I were talking about the fact that we haven’t had the levels of deployment we would like to see, because we assume that kind of data scientists would do it all and it turns out they don’t do it all. So we see more and more the adoption of data products teams, or some people call them analytics or AI products teams, but they combine data analytics and AI to accomplish something the organization wants to do with data and analytics and AI. And it may be something for customers or it may be an internal offering of some type. And this is not a new idea. A lot of the companies that are employing it are adopting it from some of the concepts in software product management with some minor changes. People need to know more about data and analytics, of course, if you’re going to be a data product manager. But I don’t know that I’ve seen yet the kind of broader infiltration, if you will, of AI into all sorts of teams within organizations. It makes sense that if our products are becoming more and more data oriented, which I think they are in every industry, that we would see the need to have AI capabilities in every sort of product development team. But I don’t know that we’ve seen that yet. I don’t know if you have a feeling about it Laks.
Laks Srinivasan:
Yeah, I think Tom, your last point to me is I think the biggest kind of insight is the difference between Ford Motor Company and Tesla, right? They both do AI, they both do software, they all have data. So to me Ford is a car company that does AI. Tesla, you could argue it’s an AI company that just happens to be on four wheels. So to me it’s again the digital native mindset. So as Tom is talking about, we are seeing vast majority of companies are treating AI either like a tactical kind of it’s part of it and data, therefore it’s a bolt-on to the existing toolkit and therefore you’re not seeing that kind of infiltration. So it to me, it speaks to some of the research that Tom and I published in a webinar with MIT. It’s clear there are some companies that are advanced. So if you look at as they mature, we are seeing that what starts out kind of in an unorganized way they hire a CDAO chief data analytics officer and they typically start to centralize build a COE or a Center of Excellence type model with a view towards not only doing AI projects, but also evangelizing and enabling various things in a way that could infiltrate into teams. But we haven’t seen yet where any of those companies have completed the journey where you actually disband the COE because you have now become Amazon, I think, Tom, am I right? Amazon and Google do not have a chief data analytics officer. I would imagine their CEO plays that role. Right?
Tom Davenport:
Yeah. It’s just so pervasive, I think, across the organization that there’s not a feeling that it’s necessary.
Laks Srinivasan:
Necessary, so to us, Simone, the model is the digital native companies, that’s a good model, but wow, the divide is so vast. Even the companies that we spoke to in our research that are generating few hundred million dollars right. Of measurable value this retailer. You know, they’re saying they have been at this for eight years, they’re barely scratching it. And it is a team of 15-20 data scientists in a Fortune 50 company. Right. And he was saying we asked him, saying, why can’t you do more? Why is AI not infiltrating more? Well, it is set up inside a merchandising department. And he said the analytics is looked at as a cost center. So the first question that is asked by the CFO is, wait a minute, you already have 25 people, why do you need to add ten more headcount? Right. So it comes at it as more of a cost center mindset rather than business acceleration mindset. This is, by the way, is the conundrum. I haven’t figured out a way to explain this. This is the challenge with AI is it is so multi-dimensional. I call the best I can explain is high dimensionality nonlinear that involves organization, leadership, culture, and let alone data and technology. And that’s what makes it so complex.
Simone Cicero:
Yeah, it seems like it’s not something you can solve with the chief AI officer. Maybe you need it as a kind of coordinator. And I think it’s a good point because when you say, for example, they see this as a cost, sometimes it seems like there is an easy perspective on AI that is, let’s use it to optimize, to solve, to automate, something like that. But then when it comes to imagining new business models, it’s very much harder.
Right. And this is not just a problem of AI, I would say. Right. In general, for companies, it’s very hard to imagine new business models and they are bureaucratically structured to execute the core business, but very rarely have the capability to really jump into new things. And Tom, you made the example of Ping An, which is I agree, it’s mind blowing. And especially I was mind blown when I was informed about the product, the insurance product that essentially liquidates 90%, let’s say, of the incidents with an AI, just interacting with an AI, showing photos of your car. And AI will make an assessment on how much money to propose you and you will take it 90% of the time without going through any process or use of resources internally to the organization. So what I would like to ask you, maybe to make it even more tangible for the companies that are listening to us at the moment, what are, let’s say, two or three incredible examples of how companies have created new business models powered by AI, which weren’t really possible, like this one we mentioned about Ping An.
Tom Davenport:
Well, I’ll start with one that’s related to what you mentioned, Simone, about Ping An. And it turns out that Ping An gets at least some of that capability from a partner company that I’ve done a lot of research on called CCC Intelligence Solutions based in Chicago and started out measuring it was something Collateral Corporation. I forget what the C stood for, the first C, but it measured kept track of values of cars so that if your car was totaled or something the insurance company would know how much to pay you. But the CEO was very technically oriented, and he started, I don’t know, maybe a decade ago, thinking that, okay, these mobile phone cameras are improving at a pretty rapid rate, and so pretty soon, we might be able to have the customer take a photo and learn what the repair of the car would cost almost instantaneously. Technology wasn’t quite ready yet. Deep learning wasn’t well established as an algorithm type yet, so they worked and worked at it and finally they were able to develop this capability in the United States. A company called USAA, which is quite progressive in terms of its technology, was the first to adopt it. And I know they’re a supplier to Ping An, but they are in addition to just using that technology, they have a network of, I don’t know, 10,000 repair shops. They work with all the original equipment manufacturers of automobiles. They work with parts suppliers. So if you’re going to facilitate the process of getting your car fixed, I actually know this because my car was recently rear ended by some woman eating a sandwich and looking down at her lunch while she should have been looking at the road. All the people that I talked to said, oh yeah, we use CCC. It makes everything the repair process and getting parts turned out to be quite problematic given the supply chain constraints that we live in today. But everything else was almost immediate and very easy because of this friction-free environment. Just a little more detail on that Ping An story. I don’t know if you want to tell, Laks.
Laks Srinivasan:
And Tom, I wish they would put AI to prevent accidents in the first place. Wouldn’t that be a better use?
Tom Davenport:
Well, I think Elon Musk would tell you that he’s done that already. But I don’t know. I’ve had a couple of Teslas and you should not believe that statement by him. In fact, I think some of the regulatory authorities are currently investigating him for making that claim.
Laks Srinivasan:
I would agree with that. And some simpler technology, Tom, and I do think I shouldn’t admit to this, but I have had my car sometimes hit the brakes when then I think it’s just much more traditional technology of just using radar to see there’s a car in front of me decelerating faster than me and it stops it. By the way, Ping An brings me memories. I’ve been in Ping An offices in Shanghai where in my prior life we actually did one of the projects in the insurance business where we brought the project to us to figure out how to target customers that go home for their, I think, annual kind of New Year holidays to sell travel insurance at the time. This one example I’ll just kind of add quickly is I saw this. This is not anybody that we have worked with but definitely piqued my interest is an Israeli company that had a lot of video kind of AI video image processing type AI capabilities, I believe is working with Volvo or one of the car companies, which I thought was fascinating. So today you want to get your car to service, you have to bring the car in. And then they left to walk around some human being. And what it does is you pull in. It has got all kinds of videos and then the AI detects various things that could be wrong, could be under the car, some axle is bent. So there are kind of new types of business models that AI will enable. And again, all around the principle that Tom very nicely wrote in the AI Advantage book. I mean, it’s all about certain tasks, narrow tasks that are getting automated. But I think humans are very much safe and it’s still having a very hard time replacing kind of humans in a hole rather than in the entire job. Just tasks.
Tom Davenport:
Yeah, that’s certainly consistent with my work in this book. Working with AI. Many, many examples of AI augmenting human labor and in some cases human labor augmenting AI. Relatively few examples of large scale automation. I would say maybe the outsourcing industry has taken the biggest hit, but even it seems to be doing fine. And within most companies, I’d say not a lot of job loss yet at all.
Laks Srinivasan:
Yeah. And Tom, you and I have talked about it a little bit. Isn’t that why kind of AI hasn’t really moved the needle on productivity at the macro level? Right? Because you’re saying, oh, it’s going to augment. People are not losing jobs because not able to automate a full job. It automates perhaps narrow tasks, some jobs it can automate. But to me, Tom would we say that for you to really get that productivity kind of step function improvement at the macro level, economy level, to me, we need essentially every company to be like Amazon and Google, isn’t it? Now we’re back to again the digital native model, AI being pervasive and it’s infiltrated to use Simone’s Word and Data Products Data Product Manager. To me, until that happens to 90% of the Fortune 500 companies, I don’t know how we are going to have impact productivity.
Tom Davenport:
Being a human, I still pulling for the humans in all of this. But we need at some point we’re spending a lot of money on AI. We need to find some way to justify it economically. Part of that is creating new business models that don’t necessarily mean replacing people, but let’s face it, people do a lot of really boring, repetitive tasks and so automating them, speeding them up, making them more efficient. I think it would be good for the economy over the long run. We might see some job displacement in the shorter run.
Luca Ruggeri:
That’s really great. I was listening to you and you made me think about before moving to the pre-crime unit of Minority Report and understanding how AI can save our lives, and before opening the chapter of building trust between humans and AI and the Robo Law and Responsibility and Liability, let me just get a little step back. I was captured by the different pace that digital native and AI native companies are demonstrating over traditional companies. But indeed there’s plenty out there of traditional companies that are evaluating how to adopt AI because of what you said, not because they want to maximize or efficientize their cost structures, the activities they go to market and their processes. So thinking about steps of adoption of a realistic and practical return of AI, so something that can be in the hands of these companies, what could be the steps or what organization traditional ones should look like when preparing to adopt AI? I was just thinking as a sub question about the big Badsworth of data and big data and data intelligence. So every company thinks that they should start from assessing data, data maturity. There is the big debate between data quantity and quality. But what’s more in terms of organizational roles of units and what steps you normally advise to a company that wants to evaluate a realistic use of AI.
Laks Srinivasan:
What I would say is if you haven’t started, start it. It could be somebody in the organization that knows something. That’s how I have seen it start. You need a spark. So if you’re not in it, don’t do strategy. This is one of those things. The strategy is actually by doing right. So I would say get started. But if you have started and you have done kind of low hanging fruits and you have done a few things, either you’re kind of in the pilot purgatory or you’re kind of done low. Hanging fruits but kind of stuck in the chasm. I think I would go back to our research that again is what we found in these companies, non-digital-native companies that are creating real value today at scale, relatively speaking is once you have gotten used to it, you have a few things going to me goes back to starts at the top is the CEO and the management team committing to AI is central to achieving their business objectives. So you have to make that commitment, you have to make that intent known. Otherwise AI will always be a bolt-on. It will live in your It world and therefore starts at the top, starts with that commitment and then idea from there would be how do you identify real high impact use cases, how do you prioritize them? And then most important from there would be once you get going with AI, it’s extremely important you measure it compared to kind of the incremental lift. So you need to bring in some way of that enablement process around it in a way that you’re monitoring, measuring value, kind of failing fast, but then investing where things are working to be able to accelerate and things like that. So to me commitment at the top and then to be able to have a process in which you have a disciplined process to be able to advance.
Tom Davenport:
Let me give an example that maybe connects the kind of high level I always say about AI. Think big but start small. Because as was saying earlier, AI is really good at tasks, individual tasks, not large scale processes or even complete jobs. So one of the companies that I’ve been working with over the last couple of years, they just changed their name. They change their name all the time but they’re now called Elevance. They’re the second largest health insurance company in the United States. They were Anthem when I was writing about them and they’re formed out of a bunch of different insurance companies and have a lot of legacy systems and so on. But they’ve decided that they want to be a platform for health and the CEO talks about it a lot. A friend of mine I used to work with, Deloitte, is the head of the platform business unit now. And so one of the things they realize that they need to do is to instead of kind of having humans approve every health treatment that they need to undergo, they want to automate some aspects of that. Well, most of the documents that they use to kind of formalize their relationship with their customers or their members as they call them, are PDF documents and the key information is stuck in a PDF. So now if you want to find out is this particular procedure covered in my policy, you have to call a call center. Somebody at the call center has to read through the PDF and try to find the answer. Takes forever. So what they’re trying to do is first take the key information out of the PDF. AI is pretty good at doing that, but it’s not a small project for all of their member agreements. Then they’ll be able to move maybe toward call center agents immediately getting the information, and then they can move to members getting the information themselves, and then they can move to AI telling them the answer to their question. But it’s a pretty long process to do all of those things, and it’s a step by step progress toward becoming a platform for health for their members.
Laks Srinivasan:
One other thing, since you’re talking about what are the tangible steps somebody could take, so clearly that for management to commit, I mean, when is the last time management or the executive teams will actually deliberately, intentionally commit to something that they don’t understand? Right? It doesn’t happen. So maybe even a prior step, which Tom and I are on the mission to do, is how do we increase that awareness? How do we increase that fluency of executive teams? Just like a digital native company. Some of these companies may have to start with that and say, we know of one company that we hope to be able to work with, is, they conducted an internal AI quotient, AQ assessment of the entire company employees, including management teams. To be able to understand what is the level of awareness and understanding. And they identified management teams. While you can do a lot of hands on a lot of self service life education available, management teams don’t learn in classes. And so therefore, how do you kind of improve that and also help them not only learn by just in classroom setting or social setting, but also how do you help them as they apply it on their jobs in a way that they build that intuition? Because executives are the ones executives don’t do things. All they do is make decisions. And for them to make decisions, they need to have intuition. And therefore, first step to all of this may be that how do you start with that at the executive levels. Then you can have them say, okay, I understand it enough to say how this could be so central to us accelerating our business. Again, Tom’s point, getting new market segments, maybe new business models, new products, getting new customer segments, all of that should be the way you start. Not, I have this data, let me build a model. I would say what’s more important in AI is how not to start, which is what 90% of the AI projects fail is because they start with data and they start with model. That’s not what we would advise to do.
Simone Cicero:
That reminds me of this idea that you start with customer experience, basically, right? So to some extent, there are lots of gaps here to bridge because, for example, you say CEO or executives in general, they need to embrace this opportunity, whole-heartedly, right? But on the other hand, it’s hard for them to understand the real possibility because they don’t know. They don’t understand what real use cases can be enabled by this. That’s the first gap. But then there is a second gap, which I think it’s also interesting to maybe touch upon as the last argument is the topic of responsibility, liabilities right. And I know that this is something that is already very well discussed in many podcasts and Q&A and so on, AI. But the point to that I would like to stress here, is as long as AI is used for, I don’t know, anticipating some of your needs, for example, proposing you a new thing to buy on Amazon or maybe as Tom was saying before helping you to find the right match on a marketplace, which are some of the uses we are more used to. But as soon as you move into real economies, like for example, health care, let’s say that for example, you use an AI to propose a certain type of care plan or something like that, there is a massive liability, right? So the question is, how does an organization understand how to manage this intricate question of liability as it integrates more AI into their process? So, as I would say, this is more like a challenge for mixed organizations, right? As they envision the possibility to really understand the use case and they’re building the technology, I’m sure that they are set to face this liability question so what is, in your experience, a good way to manage it? And maybe you can also mention regulations that you are aware of, or in general, how the policy makers are also looking into this.
Tom Davenport:
Well, I would say you have to limit your ambition. Over the last few weeks, really, we’ve seen the autonomous vehicle industry start to implode, basically the Ford autonomous vehicle subsidiary, Argo AI. Ford decided this week, we’re getting out of that. A whole variety of companies in that space are saying, well, it’s going to be a lot longer to really produce autonomous vehicles. And what Ford has decided to do, I wrote a piece about this a couple of years ago about Toyota. They’re saying, well, full autonomy is really tough. Why don’t we try for very high level of driver assistance? And so you have to, I think, temper your ambitions. Coming back to Ping An, one of their most, I think, fantastic use cases in their healthcare ecosystem is this intelligent telemedicine system called Good Doctor. And China hasn’t had enough physicians for its very large populations. So they decided, well, what if we have a sort of a triage system that can make recommendations, intelligent AI-based recommendations. Over 300 million people use this system in China. Now they’re expanding it to Southeast Asia elsewhere, Indonesia and Vietnam. But the doctor still has to make the final call on both diagnosis and treatment. That’s a matter not just of Ping An’s conservativism, but it’s in regulation. AI can do a lot of great things, but we are probably somewhat better off if we temper our ambitions for it in the short run anyway.
Laks Srinivasan:
And Tom, I’m going to use one of your quotes from your book, which is I think people overestimate the power of technology in the short term and underestimate it in the long run. That goes well with your scale, down your ambition.
Tom Davenport:
I wish I had invented that line, but it actually belongs to this guy, Roy Amara, and it’s called Amara’s Law.
Laks Srinivasan:
Yeah. And also there’s another famous quote that I can name. The CEO of a very large company, apparently. He says advisors, management teams of other companies that are thinking of adopting is with AI. It’s a long march for all the reasons we talked about. So it’s about having strategic patience and tactical impatience, I think, which is, again, back to the theme of think big but start small. And that’s why you need to have some process in a way that you’re making kind of singles and doubles, to use the baseball analogy in America. And don’t go for home runs before you fully understand because there are so many examples what Microsoft shut down that you could upload a photo. It recognizes something about your gender, race or whatever, and they shut that down in about five minutes. Right? So just be careful because AI is very powerful, creates a lot of value. In the wrong hands, it can create a mess. And if you’re a CEO, a board member, you’re a management team or officer of a company, you don’t know it, but you’re owning financial reputational, legal risk today because I’m sure there’s AI in some form or the other internally going on. Another thing would be just because you can just don’t do it, understand it. And just like with any design, thinking with any other technology and project, think through all the impacts. And I think there is a lot of kind of movement going on. And in our podcast, the ROI Playbook we recently, which we released yesterday, we had Jack Hanlon, who’s a VP of from Reddit and we asked him, saying what worries him most is he’s got so much data through Reddit, he can do a lot of things, but it’s not good. So in essence, think about what is good, not just what would add to your bottom line. Could also be another way. Again, bring it back to our institute’s original manifesto. Tom wrote is it’s not about just economic return. Also think about social return. So combining those two will give you some idea of how to manage risks and how to be responsible.
Simone Cicero:
So first of all, we said organizations need to tackle AI both at centralized level, let’s say. So at the core, at the top, I would say, but also in the periphery. So we are also envisioning that as AI becomes more manageable in terms of as a technology more accessible teams may have to embed some kind of AI capability. So which is a very interesting point. Then I think also another very important point we raise is that execs should be able to wholeheartedly embrace and invest into AI but there is this gap to fill which is they have to see the possibility in terms of new use cases which it’s really a conundrum because probably you have to rely on external knowledge or maybe hire some consultants or maybe invest heavily into building capabilities internally before you start to even see the possibilities. Which is another very interesting point here. Also, another thing that came out is it’s a really long run because only if you scratch the surface and then you go deeper and then you really understand what kind of use cases you can implement. At the end of the day you may also, especially if you deal with real economies or tangible economies you may end up in hitting these liability questions that are likely. I would say. I was thinking about this and I was thinking when are we going to solve this liability issue? And yeah, I tended to think about we’re going to solve the day, we’re going to consider AI as humans when we have AGI and then God knows what happens when we have AGI.
Tom Davenport:
All bets are off when we have AGI.
Laks Srinivasan:
Right.
Simone Cicero:
So at the moment the gospels out of the shell then we’ll see. So this is really something that stick to my mind. So can you share maybe a couple of breadcrumbs for our listeners? So things that you want to share with them? It’s not going to be necessarily something about AI but I’m sure that you have something very interesting about this. It can be a movie, song or whatever really something that you believe it’s important for our listeners to catch up with as a piece of work.
Laks Srinivasan:
I know Simone wants me to publicly admit I don’t have a life. I don’t do anything interesting. But no, I’ll tell you one thing I am thinking about. I used to be a volunteer firefighter which I trained, went to fire school. Believe it or not, there is a fire school that you learn and so I was just thinking about that and I’ve stopped kind of being able to do because I was traveling all the time. But with COVID now we’re all working from home and I’m much more accessible because I live in a town of 10,000 people and it’s all volunteers. I’m about to go back and sign up again which I think is going to be interesting. However, there’s something that triggered which I think could be interesting is that I was just thinking of difference between what I can learn from firefighting and AI. Right, always I’m thinking about AI is how many times they take firefighters new and they just send them to fire never happens. I went to school, but I had to go through at least three months of drills every Monday night on makeup fires. They train you and then eventually you go fight fires. You make mistakes and then that’s how you learn. So the question is, this is what we are dealing with for executives. So we have to figure out a way of not just classroom knowledge, which is what they can go to MIT and take class, but the key is, how do you so we are working on some projects which is drawing on this fire analogy. We’ll hope to kind of get that and then try that out in some companies. So that’s coming.
Simone Cicero:
Thank you so much for shedding some light on something so important as fire volunteering for fire control, fire department. So thank you so much.
Tom Davenport:
Well, I’ll relate this to how I spend my time. I don’t do anything as heroic as fighting fires, but by profession, I’m a content creator. My son is a TV comedy writer. My daughter in law is a movie writer. My other son writes AI related articles. So my whole family is in the content creation business, and I think it’s going to be really interesting over the next few years to see does AI take a lot of that over? I just wrote a Harvard Business Review article, not yet published about these generative AI systems. And the first paragraph I had written by GPT-3 to introduce the topic and it was pretty good. Thought of some things that I wouldn’t have thought of. It wasn’t perfect as we were saying, it needs a human at the beginning to create the prompt and then a human at the end to edit it. But it really accelerates the process dramatically. So I think for all of us I recommended, my son just had our first grandchild, and he had to send a lot of thank you notes. And I said, if you’re having a hard time, why don’t you use some of these AI systems to generate some new ideas for your thank you notes? And he said it worked pretty well. So I think we’re all going to be living in a very different world of content creation. It’s going to be interesting and maybe scary. I’m also wondering how the hell am I going to grade my students papers if they’ve been written with AI? And will I even be able to tell?
Laks Srinivasan:
Tom, wouldn’t you be able to have AI algorithms grade as well?
Tom Davenport:
I hope so. It’s interesting we’ve had algorithms that would identify plagiarized material. Maybe we’ll have algorithms that identify this was created by another algorithm, but it’s an ongoing war, I guess, between the good guys and the bad guys.
Simone Cicero:
That’s good because this week I’m testing Lex.com, this new tool that Nathan Bassett just released last week from Every. And at the end of the presentation, they say occasionally this tool may be plagiarizing someone. So you have to check. How I’m supposed to check all of the database that has been trained to use this stuff? So it’s really crazy. It’s a good example of the disruptions and the complexities that we’re going to have by leveraging on those great powers. And really, as the movie goes, great power comes with great responsibility. So that’s it. So thank you so much. It was an amazing conversation. First of all, thank you, Laks and Tom, I hope you also enjoyed the conversation. Very much. Thank you so much. And thank you, Luca, for sparring partnering with me on this.
Luca Ruggeri:
Thank you. Thank you, It has been a real pleasure.
Simone Cicero:
And for the listeners, please go on the boundaryless.io/resources/podcast website. There you can find all the show notes and the transcript and all the links, all the things that we mentioned today. And let’s catch up as you listen to this podcast. And please remember to think Boundaryless.